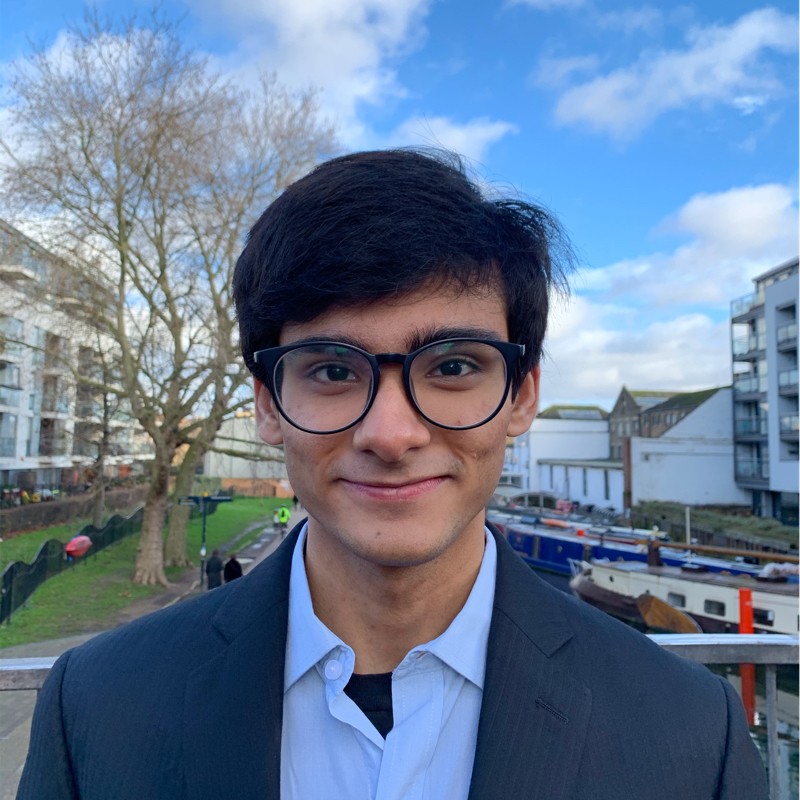
Written by
Shahzeb Khan
Our CTO, Julio Amador, envisions a world where customs brokers trust numbers when making risk decisions.
Statistics are at the core of any Machine Learning (ML) application. According to Deep Learning theory, an algorithm will have to learn about 5,000 different examples of 1 variable to achieve acceptable performance—and 10 million to exceed human performance.
In this article, I would simplify our choices into 2 categories: repetitive and predictive. The repetitive decisions are the ones we make automatically without much consideration. For example, when making coffee, we do not think too deeply about what beans to use or the temperature, as we have done the same tasks hundreds of times.
However, say you wake up late or have a vast overnight project that breaks your routine. This disruption forces us to decide when is the best moment to drink coffee which depends on several factors: our last meal/drink, the next activity, the facilities available, our next meal, and any other factor that could affect our decision. To conclude, we assign probabilities to each of these factors based on what we know, i.e. we make a prediction. These are ‘predictive’ decisions, and we make hundreds of them throughout the day.
An algorithm is a series of steps a computer takes to complete a task. Computers even use algorithms to come to decisions when things are unclear. Machine Learning is the process through which computers learn the steps of these algorithms by finding patterns and probabilities of scenarios occurring in historical data. For example, suppose a computer scientist wants to teach a computer to identify dogs. In that case, they have to give a computer thousands of examples of dogs and not dogs—the historical data. Each dog example is different, and the computer looks through all the samples to find patterns which it can use to build an algorithm to decide whether a particular picture is a dog or not.
We can’t teach a computer to decide for a person when is the best moment to drink coffee. It is too complex. But computers can recognise patterns in data. So instead, let's ask a computer to decide how to make good coffee. It will look at the historical data (different examples of how to make coffee), find all the patterns between the samples, and merge them to decide the best way to make coffee. At the same time, if you were to ask a human the same question, they would either give their own opinion, which might be inaccurate or have to research online and consult experts, which would take significantly longer. The advantage is that computers are faster to find such patterns based on the historical database or “knowledge”.
A well-designed ML algorithm with a balanced and unbiased training set (historical data) will have accurate probabilities for each variable to decide. Business-applied statistics for decision-making is a complicated way of saying, “in our company, we use Machine Learning''. However, ML is best at repetitive tasks but struggles with predictive ones. Therefore, human decision-makers cannot wholly depend on the output of computers but instead use it to inform their decision-making and trust that the numbers given by Machine Learning accurately represent the data.
Read more about what else is happening at Sifty.
Enter your email, and our team will reach out to you shortly.